Dust storms are not only a nuisance for anyone trying to keep their house spick and span, they also pose a very real health hazard and are a major ecological concern. Respiratory problems caused by breathing in dust and other airborne particles are one of the main causes of death worldwide. To make matters even worse, dust particles, which travel freely from country to country and from continent to continent, can spread pathogens, possibly contributing to the outbreak of pandemics. Moreover, dust clouds have a hugely significant impact on the climate: They absorb and distribute the sun’s rays, thereby altering the Earth’s temperature, and they also affect the properties of clouds and patterns of rainfall.
Usually, dust storms form in arid areas, such as the Negev, the Arabian Peninsula, the Sahara and the North American and Asian deserts. The wind kicks up tiny particles from the ground and, while the larger sand particles sink close to where the storm formed, the smaller dust particles can be blown hundreds or even thousands of kilometers away. Having an early warning for waves of dust could help protect vulnerable populations and prevent the destruction of crops – and, as a bonus, save us from pointlessly cleaning our homes. But the rapid development and spread of these storms, coupled with the fact that they stretch over massive areas, makes it difficult to predict when, where and how badly they will strike.
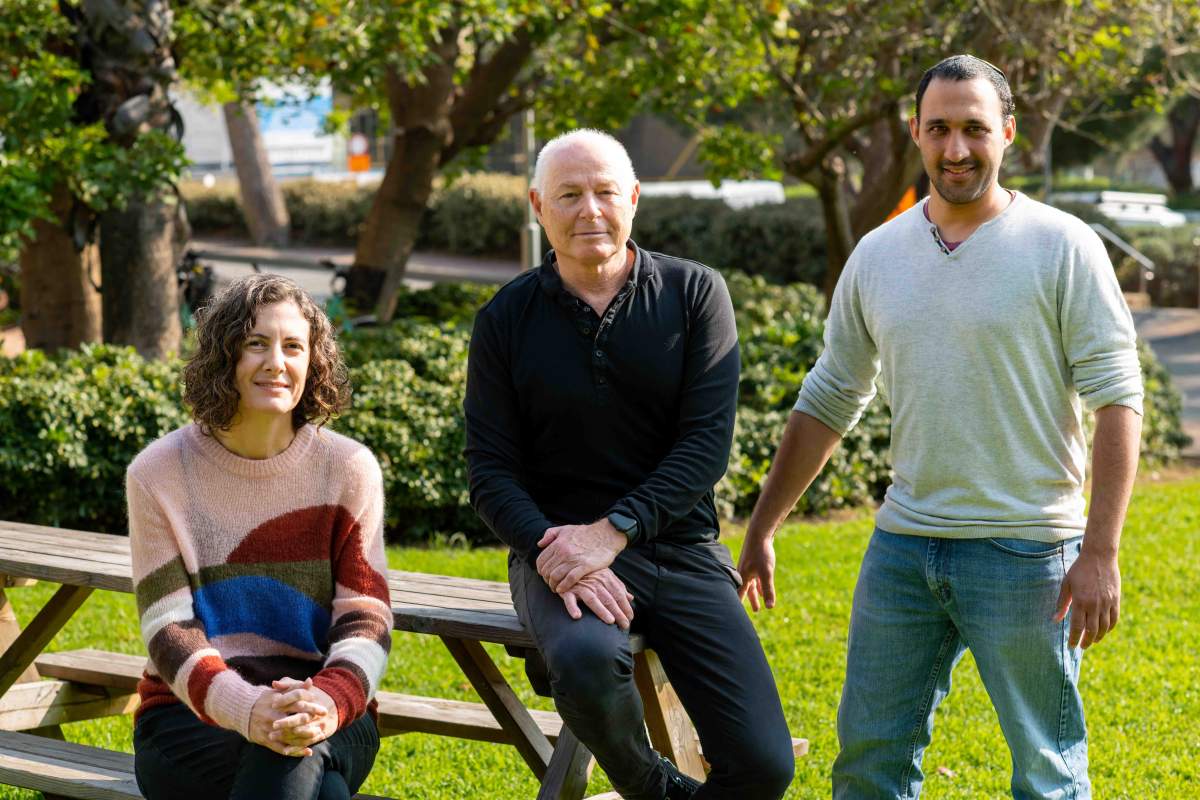
A recently published study by Dr. Ron Sarafian, Dori Nissenbaum and Prof. Yinon Rudich from the Earth and Planetary Sciences Department at the Weizmann Institute of Science brings a breakthrough in dust-storm forecasting. The study, published in npj Climate and Atmospheric Science, was written in collaboration with Dr. Shira Raveh-Rubin, also from the same department at Weizmann.
Originally, the researchers hoped to use knowledge garnered in the field of computer vision. Since meteorological data of a dust storm can be displayed as a series of satellite images, they thought that an artificial neural network would be able to “learn” the patterns governing the spread of storms – just as these networks have learned to recognize videos of various animals or objects.
""The network trained on data from Israel can, with a few adjustments, forecast dust storms elsewhere in the Middle East and even across the world"
Their hopes, however, were only partially realized. A regular image is comprised of just three primary colors, with a fair amount of overlap between them. Meteorological “images,” however, are made up of no fewer than 60 variables: temperature data, humidity, windspeed and so on. In addition, while computerized vision systems rely on machine learning based on archives of millions of images, there were precious few images available for an artificial neural network tasked with identifying dust storms: Israeli researchers have at their disposal just 60,000 of these meteorological “movies,” after collecting detailed data from satellites and ground stations for around two decades. In this relatively limited collection, it is rare to find multiple instances of a dust storm forming in the same location.
In such cases, any artificial neural networks trying to learn the patterns governing the formation of dust storms in Beersheba, for example, could suffer from what’s known as “overfitting.” In other words, they might formulate patterns based on limited circumstances and reach incorrect conclusions when new, not-yet-learned conditions are discovered.
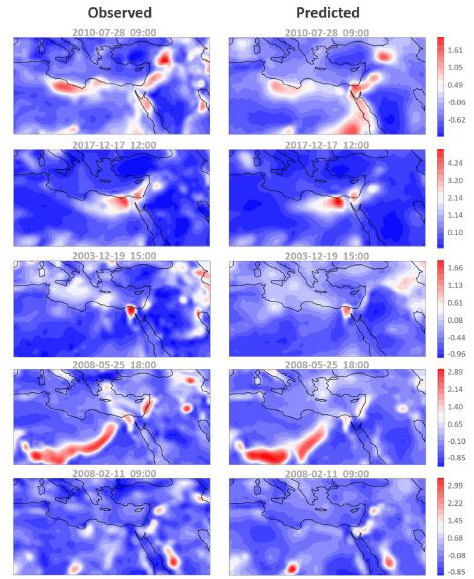
To their surprise, the researchers discovered that forecasting could be improved by making life harder for the artificial neural network. They tasked the network not only with learning when a dust storm was expected to reach a certain point, but also to handle an auxiliary problem: keeping track of the much larger area in which the dust is dispersed. For example, in order to predict when a dust storm would likely hit Beersheba, the network learned how badly the storm had affected Lebanon. Using this approach, the network had access to a much larger collection of data, from which it could also learn about the physical and meteorological circumstances by which dust is spread.
Making use of data collected from all of Israel’s meteorological stations over the past 20 years, the researchers showed that during the dust-heavy winter and spring, they could successfully forecast more than 80 percent of dust storms 24 hours ahead of time, and around 70 percent, 48 hours ahead of time. Most of the incidents that the system did not predict were storms that developed rapidly in a localized area, which makes it difficult to collect regional data that can help predict them.